Development and validation of risk-adjustment models for elective, single-level posterior lumbar spinal fusions
Introduction
Following the passage of the Affordable Care Act (ACA) in 2010, there has been increased focus on delivering quality care at a lower cost, driving the shift from traditional fee-for-service reimbursement structure to a pay-for-performance model, especially for orthopaedic procedures (1-8). To succeed in these programs and maximize value for the patient, orthopaedic providers require data-driven tools to efficiently allocate resources before, during, and after the target procedure. Effectively managing patient care and optimizing long-term outcomes must remain the main focus for orthopaedic surgeons. Risk stratification models can help ensure good patient care and allow for appropriate reimbursement for this clinically complex patient population.
Risk stratification accomplishes the above objectives as it involves an important trade-off between data collection burden and the ability to identify and manage high-risk patients. There are numerous risk-adjustment models used to calculate appropriate capitated payment for services (9-11). However, several studies have demonstrated the limitations of claims-based risk-adjustment models that are focused on preventing short-term complications (9-12). Only a handful of models such as the America Joint Replacement Registry’s 90-day infection/1-year revision risk calculator for total hip/knee arthroplasty (THA/TKA) patients utilize clinical data (13,14). The need for additional orthopaedic-centered risk-adjustment models is evident.
Over the past two decades, the volume of elective, single-level, posterior lumbar spinal fusions (PLSFs) performed has increased by 137%, targeting the procedure for bundled care models (15). There are many studies evaluating the link between patient characteristics and provider factors (i.e., surgeon volume, operative technique) on short-term outcomes following PLSF (16-18). However, there is a paucity of literature that seeks to identify a limited-set of “most-predictive” risk factors that can be practically implemented.
The goal of this study was to develop two sets of risk-adjustment models specific to 30-day severe adverse events (SAEs) and unplanned readmission following elective PLSF using a large, nationally representative clinical database. A second aim was to provide a basis for spine surgeons and hospitals to make informed decisions when constructing clinical data-driven risk-adjustment models for PLSF to balance the trade-off between improved risk stratification accuracy versus clinical data collection burden.
Methods
Study population
CPT codes (22612, 22630, 22633) were used to identify individuals 17 years or older who underwent single-level PLSF with or without use of an interbody device within 2011–2014 American College of Surgeons National Surgical Quality Improvement Program (ACS-NSQIP) data (19). Those with additional CPT codes not corresponding to single-level PLSF were removed, as were patients with pre-operative wound infection, disseminated cancer, or those who underwent emergent spine surgery. Procedures from 2011 to 2013 constituted our derivation cohort while procedures from 2014 were used as a validation cohort for our risk-adjustment models.
Outcome measures
The 30-day SAE and 30-day unplanned readmission were the two outcome variables of interest; 30-day SAE included death, myocardial infarction, cerebrovascular accident, renal failure, pulmonary embolism, venous thromboembolism, sepsis, septic shock, unplanned intubation, paraplegia, deep wound infection, organ/space infection, and return to operating room.
Patient characteristics
Table 1 displays patient-level covariates considered for our PLSF model analyses. These included: age, American Society of Anesthesiologists (ASA) physical status classification, comorbidities, laboratory values, vital signs-based comorbid conditions and intraoperative variables. Vital signs-based comorbid conditions included systemic inflammatory response syndrome (SIRS) and septic shock. Intraoperative variables were comprised of operating time and total hospital length of stay. Laboratory values were denoted as normal or abnormal based on accepted norms (20). Age was also reported as a quadratic with the assumption of improved fit. More information regarding each variable can be found in the ACS NSQIP Participant Use Data File (PUF) (21). A category for patients with missing laboratory data was created, and the outcomes for this group were compared and pooled into the reference group (22).
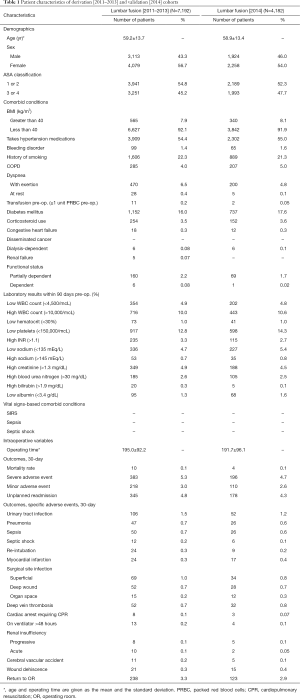
Full table
Bivariate analysis
Bivariate analysis of risk factors was conducted for each of the outcome variables. Table 2 displays odds ratios of the statistically significant patient-level covariates for the derivation cohort and the validation cohort.
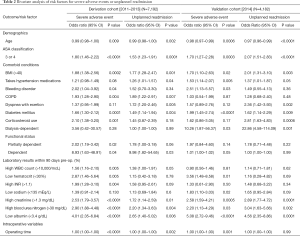
Full table
Specification of full risk-adjustment models
Variables with a P value ≤0.10 in bivariate analysis were included in separate stepwise regression models for 30-day SAE and 30-day unplanned readmission. All variables that entered and exited the model with a P value of 0.10 were retained, and those that were statistically significant are shown in Table 3.
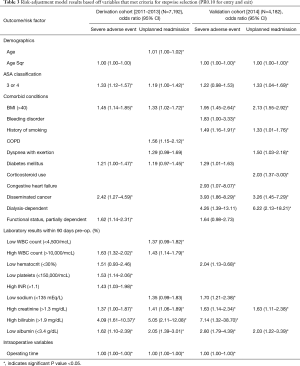
Full table
Evaluation and validation of full risk-adjustment models
The models were evaluated and compared for predictive performance (discrimination) and goodness-of-fit (calibration) (Table 4). C-statistics described the predictive value of each model while results from the Hosmer-Lemeshow test were used to assess calibration of the models. Derivation and validation models were evaluated and compared against each other to assess overall model performance and consistency.
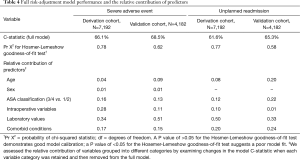
Full table
Predictor contribution to full risk-adjustment models
We determined the relative contribution of each variable to each model by sequentially removing variables one at a time (Table 4). The exact contribution was calculated by analyzing the change in the model log-likelihood value when a given variable was removed. Variable categories included age, sex, ASA classification, comorbid conditions, laboratory values and intraoperative variables.
Limited risk-adjustment models and prediction value
All models were analyzed using fewer covariates and built up sequentially to the full model to understand the predictive value of models without the full complement of clinical data (Table 5). Covariates were added back starting with ASA classification and progressing through the addition of comorbid conditions, laboratory values, age and intraoperative variables until the full model was formed. Prediction performance was based on C-statistics and calculations of the continuous net reclassification improvement [NRI (>0)] (23).
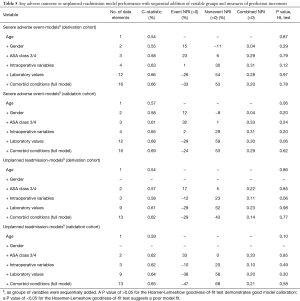
Full table
Results
Patient characteristics, intra-operative variables, and 30-day outcomes
The derivation and validation cohorts consisted of 7,192 and 4,182 patients respectively and were similar in terms of demographics, characteristics, clinical comorbidities, pre-operative laboratory data, and intraoperative variables (Table 1). There were no statistically significant differences in post-operative 30-day outcomes.
Bivariate analysis
Both validation and derivation cohorts identified age, ASA 3/4, BMI >40, hypertension, bleeding-causing disorders, diabetes mellitus, corticosteroid use, dependent functional status, low hematocrit, high INR, high creatinine, high BUN, and low albumin as factors associated with SAEs or unplanned 30-day readmissions (P<0.05) (Table 2).
Multivariate risk-adjustment models
Stepwise logistic regression in the derivation cohort yielded models with 12 and 11 significant independent predictors of 30-day SAEs and unplanned readmission (Table 3) while 12 and 10 predictors were generated for the validation cohort. The derivation and validation models for 30-day SAEs both identified age, BMI >40, and high INR as significant predictors while those for 30-day unplanned readmission identified ASA 3/4 and BMI >40 (Table 3).
Evaluation of risk-adjustment model performance
Model performance was similar for the derivation and validation cohorts (Table 4). The risk models were more predictive of 30-day SAEs than for 30-day unplanned readmission. The C-statistics for models predicting 30-day SAEs were 66.1% (derivation cohort) and 68.5% (validation cohort), while those for unplanned readmission were 61.6% (derivation cohort) and 65.3% (validation cohort). All models demonstrated good calibration and fit (P≥0.58 for all).
Relative contribution of predictors to risk-adjustment models
Table 4 displays the risk-adjustment contribution to the C-statistic of each predictor group. Intraoperative variables, laboratory values, and comorbid conditions explained >75% of the variation in 30-day SAEs for both the validation and derivation cohorts. For 30-day unplanned readmission, ASA class, laboratory values, and comorbid conditions accounted for >80% of model risk prediction in the derivation cohort while age provided a 20% contribution in the validation cohort.
Table 5 demonstrates the change in performance of the risk models with sequential addition of each of the variable group (comorbid conditions, labs, intraoperative, age, gender, ASA 3/4) starting with comorbid conditions. This process was also repeated but in reverse order. For the 30-day SAE derivation and validation models, four variables (age, gender, ASA 3/4, operative time) were sufficient to achieve a C-statistic within 4 percentage points of the full model (16 variables). These four variables were also sufficient to achieve a C-statistic within 2 percentage points of models in using only labs, comorbidities, and operative time (derivation model C-statistic: 0.63; validation model C-statistic: 0.65) (Table 5). Among the aforementioned four variables, ASA 3/4 and operative time improved risk prediction (NRI >0) via reclassification of events and nonevents while gender only improved reclassification of nonevents (Table 5). Among the 30-day unplanned readmission models, three variables (age, ASA 3/4, operative time) were sufficient for achieving a C-statistic within 4 percentage points of the full model (13 variables). These three variables also achieved a C-statistic within 0–3 points of models using only labs, comorbidities, and operative time (derivation model C-statistic: 0.58 validation model: 0.62) (Table 5). In both derivation and validation models, operative time improved risk prediction (NRI >0) via reclassification of nonevents.
Discussion
As the US healthcare landscape continues to shift towards a value-based payment system, the need for adequate risk-adjustment in making meaningful outcomes comparisons has increased. The great variability within the patient population must be taken into account to ensure hospitals are not penalized for treating more complicated patients. Refining our understanding of the most predictive risk factors of adverse events can guide efforts to modify these factors pre-operatively. We created a set of PLSF risk-adjustment models to help address these issues for a high-volume procedure using a large, nationally representative database. The models for 30-day SAEs and for 30-day unplanned readmission aim to identify the most predictive clinical variables for risk adjustment in PLSF patients and assess the predictive ability of specific combinations of variable groups (13).
Performance of full and limited set risk models
Our model results indicate that it is possible to provide acceptable risk-adjustment for SAEs and unplanned readmission within thirty days following PLSF using only the most predictive variables. The full derivation and validation models provide moderate patient-level discrimination (C-statistic >0.65) for SAEs and unplanned readmission across the full spectrum of patient risk. Previous work using the ACS-NSQIP database to develop and validate risk-adjustment models for HFR, THA, and TKA noted similar discrimination for SAEs (C-statistic >0.60) compared to the model we present (14), and other specialties have likewise noted acceptable predictive ability for morbidity (C-statistic >0.70) in risk-adjustment models for five common general surgery procedures.
In contrast, we found laboratory values comprised seven of the 10 variables with the highest standardized coefficients for risk of SAE/unplanned readmission in PLSF patients. Multiple database and retrospective studies have noted the importance of nutritional status, liver disease, and pre-operative infection on short-term complication risk after lumbar and cervical fusion (24-26). The remaining three variables of the 10 most predictive in our models were clinical comorbidities (such as diabetes, COPD, BMI >40), which is consistent with the literature (13,24).
Our analysis of the relative contribution of each variable group revealed that age, ASA class 3/4, and gender collectively contributed 21–23% explanatory value to our four PLSF models, although this is less than the 38–55% explanatory value contributed in TKA, THA, and HF models (14). However, another study found a similar discrimination as compared to the full models, albeit with different variables (13). In our limited-set models, the comorbid condition, laboratory value, and intraoperative variable groups (Table 5) achieved discrimination within 1 percentage point of the full models.
Implications for risk models in spine surgery
The implications of our findings are best understood when taking into consideration both data collection burden and unexplained variation in 30-day SAEs/readmissions for spine surgery. Hospitals are generally unable to leverage existing healthcare information technology infrastructure to reduce duplication of clinician data collection efforts and respondent burden (27). Thus, most risk-adjustment models for readmission and mortality have been based on claims data because they are easily available and provide a longitudinal view of outcomes and resource utilization (27). Limitations of claims data include incorrect recording of diagnoses, under-coding of clinical complications, and can lead to bias and inadequate risk-adjustment. However, they must be weighed against the labor and financial costs of clinical data collection. Since claims provide a total cost of care perspective, the ideal data set would integrate claims and clinical data to provide the most accurate longitudinal view of outcomes and cost.
Our analysis suggests that laboratory values and comorbid conditions individually provide the most explanatory value to the PLSF model’s predictive ability. Age, gender, and operative time are readily available in the HER, facilitating input into a clinical registry/risk model. In contrast, collection of comorbid conditions is challenging because most registries require a trained clinician to extract these data from the chart. As was shown in our analysis of limited set PLSF models, the models with laboratory values, clinical comorbidities, and operative time achieved a 2–3 percentage point higher discrimination as compared to the model with age, gender, ASA class, and operative time.
Our full and limited set PLSF models for SAEs and unplanned readmission were adequate (C-statistic >0.65) but less robust than other published ACS-NSQIP models like those predicting 30-day mortality (C-statistic >0.90) (13,14). First, we recognize that SAE includes 15 different adverse events and that unplanned readmission in PLSF patient can be due to numerous reasons. One explanation for only moderate patient-level discrimination is that individual adverse event and readmission types are affected differently by different variables. We hypothesize that other important data elements from literature such as provider-related factors (surgical approach, surgeon volume, hospital quality performance, perioperative protocols), patient-reported outcomes (PROS, pre-operative pain, function, quality of life, mental health status), and psychosocial factors (socioeconomic status, insurance status, home support) may all play important roles in addressing the unexplained variation in a patient’s risk for 30-day complications, as shown in a recent model for six different conditions/procedures (including THA/TKA) that demonstrated a 45-point increase in explanatory value of the overall model via the addition of sociodemographic status (SDS) (19).
There are several limitations inherent to large databases, one of which is missing data. An analysis of 80,000 spine surgery patients in the ACS-NSQIP found that 5% were missing demographic data, 72% were missing comorbidities, and 80% were missing at least one laboratory value (23). The authors developed three different approaches for handling the missing values leading to differences in variables that entered risk models and beta coefficients for those variables. In our analysis, we excluded patients missing critical data elements (such as age, gender, ASA class, operative time). Since ~70% of our PLSF cohort were missing at least 1 laboratory value, we followed a pooled approach for replacing the missing values similar to that of other similar published analyses. A second limitation is that the ACS-NSQIP focuses on general surgery cases and is built for optimizing care of those procedures. A spine specific registry that captures more relevant perioperative clinical data and extends the post-procedure period to 90 or 180 days may prove more beneficial for PLSF patients (13,14). Another limitation of our work is that we only took patient-specific (e.g., BMI) or operating room-specific (e.g., operating time) risk factors into account. Additional risk factors, such as insurance status and ethnicity/race, may also impact our findings; while these are not modifiable by the surgeon preoperatively, such knowledge could assist surgeons in delivering care. Future work can seek to analyze the impact of these factors on 30-day SAEs and unplanned readmission in elective, single-level PLSF cases. Lastly, we are inherently limited by the accuracy of the data entered into ACS NSQIP. However, previous spine research has suggested that ACS NSQIP is better suited for adverse-event studies compared to large claims databases [e.g., nationwide inpatient sample (NIS)] (28).
In summary, the goal of this study was to improve the design of risk-adjustment models in spine surgery, including those used prospectively in healthcare delivery and retrospectively in alternative payment models. We believe our analysis demonstrates the important trade-offs physicians, hospitals, and payers/employers must take into account when deciding which data to include in risk models for high-volume, relatively homogenous procedures such as PLSF. Future work can evaluate whether alternative methods of developing risk-adjustment models—such as those created by machine learning—perform better than more traditional statistical approaches—such as those presented in the current study.
Acknowledgements
None.
Footnote
Conflicts of Interest: The authors have no conflicts of interest to declare.
Ethical Statement: ACS NSQIP is a publicly available database with no patient identifiers; thus, IRB approval is not needed for its use.
References
- Bhattacharyya T, Freiberg AA, Mehta P, et al. Measuring the report card: the validity of pay-for-performance metrics in orthopedic surgery. Health Aff (Millwood) 2009;28:526-32. [Crossref] [PubMed]
- Bhattacharyya T, Mehta P, Freiberg AA. Hospital characteristics associated with success in a pay-for-performance program in orthopaedic surgery. J Bone Joint Surg Am 2008;90:1240-3. [Crossref] [PubMed]
- Bosko T, Dubow M, Koenig T. Understanding Value-Based Incentive Models and Using Performance as a Strategic Advantage. J Healthc Manag 2016;61:11-4. [Crossref] [PubMed]
- Burwell SM. Setting value-based payment goals--HHS efforts to improve U.S. health care. N Engl J Med 2015;372:897-9. [Crossref] [PubMed]
- Lansky D, Nwachukwu BU, Bozic KJ. Using financial incentives to improve value in orthopaedics. Clin Orthop Relat Res 2012;470:1027-37. [Crossref] [PubMed]
- Wei DH, Hawker GA, Jevsevar DS, et al. Improving Value in Musculoskeletal Care Delivery: AOA Critical Issues. J Bone Joint Surg Am 2015;97:769-74. [Crossref] [PubMed]
- Bozic KJ, Ward L, Vail TP, et al. Bundled payments in total joint arthroplasty: targeting opportunities for quality improvement and cost reduction. Clin Orthop Relat Res 2014;472:188-93. [Crossref] [PubMed]
- Iorio R, Clair AJ, Inneh IA, et al. Early Results of Medicare's Bundled Payment Initiative for a 90-Day Total Joint Arthroplasty Episode of Care. J Arthroplasty 2016;31:343-50. [Crossref] [PubMed]
- Buchner F, Goepffarth D, Wasem J. The new risk adjustment formula in Germany: implementation and first experiences. Health Policy 2013;109:253-62. [Crossref] [PubMed]
- Chang RE, Lai CL. Use of diagnosis-based risk adjustment models to predict individual health care expenditure under the National Health Insurance system in Taiwan. J Formos Med Assoc 2005;104:883-90. [PubMed]
- Donato R, Richardson J. Diagnosis-based risk adjustment and Australian health system policy. Aust Health Rev 2006;30:83-99. [Crossref] [PubMed]
- Lawson EH, Louie R, Zingmond DS, et al. A comparison of clinical registry versus administrative claims data for reporting of 30-day surgical complications. Ann Surg 2012;256:973-81. [Crossref] [PubMed]
- Dimick JB, Osborne NH, Hall BL, et al. Risk adjustment for comparing hospital quality with surgery: how many variables are needed? J Am Coll Surg 2010;210:503-8. [Crossref] [PubMed]
- Schilling PL, Bozic KJ. Development and Validation of Perioperative Risk-Adjustment Models for Hip Fracture Repair, Total Hip Arthroplasty, and Total Knee Arthroplasty. J Bone Joint Surg Am 2016;98:e2. [Crossref] [PubMed]
- Rajaee SS, Bae HW, Kanim LE, et al. Spinal fusion in the United States: analysis of trends from 1998 to 2008. Spine (Phila Pa 1976) 2012;37:67-76. [Crossref] [PubMed]
- Lee NJ, Kothari P, Phan K, et al. Incidence and Risk Factors for 30-Day Unplanned Readmissions After Elective Posterior Lumbar Fusion. Spine (Phila Pa 1976) 2018;43:41-8. [Crossref] [PubMed]
- Pugely AJ, Martin CT, Gao Y, et al. Causes and risk factors for 30-day unplanned readmissions after lumbar spine surgery. Spine (Phila Pa 1976) 2014;39:761-8. [Crossref] [PubMed]
- Su AW, Habermann EB, Thomsen KM, et al. Risk Factors for 30-Day Unplanned Readmission and Major Perioperative Complications After Spine Fusion Surgery in Adults: A Review of the National Surgical Quality Improvement Program Database. Spine (Phila Pa 1976) 2016;41:1523-34. [Crossref] [PubMed]
- Nagasako EM, Reidhead M, Waterman B, et al. Adding socioeconomic data to hospital readmissions calculations may produce more useful results. Health Aff (Millwood) 2014;33:786-91. [Crossref] [PubMed]
- Ayers DC, Fehring TK, Odum SM, et al. Using joint registry data from FORCE-TJR to improve the accuracy of risk-adjustment prediction models for thirty-day readmission after total hip replacement and total knee replacement. J Bone Joint Surg Am 2015;97:668-71. [Crossref] [PubMed]
- Hammill BG, Hernandez AF, Peterson ED, et al. Linking inpatient clinical registry data to Medicare claims data using indirect identifiers. Am Heart J 2009;157:995-1000. [Crossref] [PubMed]
- Tabak YP, Johannes RS, Silber JH. Using automated clinical data for risk adjustment: development and validation of six disease-specific mortality predictive models for pay-for-performance. Med Care 2007;45:789-805. [Crossref] [PubMed]
- Basques BA, McLynn RP, Fice MP, et al. Results of Database Studies in Spine Surgery Can Be Influenced by Missing Data. Clin Orthop Relat Res 2017;475:2893-904. [Crossref] [PubMed]
- Fu MC, Buerba RA, Grauer JN. Preoperative Nutritional Status as an Adjunct Predictor of Major Postoperative Complications Following Anterior Cervical Discectomy and Fusion. Clin Spine Surg 2016;29:167-72. [Crossref] [PubMed]
- Kong CG, Kim YY, Ahn CY, et al. Diagnostic usefulness of white blood cell and absolute neutrophil count for postoperative infection after anterior cervical discectomy and fusion using allograft and demineralized bone matrix. Asian Spine J 2013;7:173-7. [Crossref] [PubMed]
- Liao JC, Chen WJ, Chen LH, et al. Complications associated with instrumented lumbar surgery in patients with liver cirrhosis: a matched cohort analysis. Spine J 2013;13:908-13. [Crossref] [PubMed]
- Gliklich RE, Dreyer NA, Leavy MB. editors. Registries for Evaluating Patient Outcomes: A User's Guide. AHRQ Methods for Effective Health Care. Rockville (MD): Agency for Healthcare Research and Quality (US), 2014.
- Somani S, Di Capua J, Kim JS, et al. Comparing National Inpatient Sample and National Surgical Quality Improvement Program: An Independent Risk Factor Analysis for Risk Stratification in Anterior Cervical Discectomy and Fusion. Spine (Phila Pa 1976) 2017;42:565-72. [Crossref] [PubMed]